A Data-Driven Analysis of Low-Cost Digital Offerings in 2024
A Data-Driven Analysis of Low-Cost Digital Offerings in 2024 - Low Cost Machine Learning Platforms Under $50 Monthly in Q4 2024
The availability of affordable machine learning platforms under $50 a month continues to expand in Q4 2024, with new players entering the field and established ones enhancing their offerings. A notable trend this quarter is the integration of generative AI features, making it possible to customize and refine language models at a lower cost. This opens doors for users to experiment with these advanced techniques without needing expensive resources. Platforms such as BigML and KNIME are gaining notice for their user-friendly workflows that cater to a wide spectrum of data science skills. However, it's crucial to thoroughly assess the different platforms as their strengths in supporting model development can vary considerably. The expanding use of data-driven insights necessitates these economical, yet powerful, tools for broader adoption of machine learning across various domains.
The landscape of machine learning platforms has shifted, with a growing number of options available for less than $50 per month. It's intriguing to see that these budget-friendly platforms have begun to integrate data visualization capabilities directly into their workflows. This simplifies the process of interpreting model results, keeping everything within a familiar environment.
Another fascinating development is the increased prevalence of automated machine learning features. This trend is democratizing the field by enabling individuals without extensive coding backgrounds to build models. It's lowering the barrier to entry for many who might otherwise find it daunting.
Furthermore, community aspects are emerging, with platforms fostering collaboration through shared datasets, models, and algorithms. This not only speeds up development for individuals but also helps create a sense of shared learning across the user base.
Interestingly, the performance of these lower-cost options can sometimes rival that of premium platforms, particularly on specific tasks. This has us questioning whether the high price tags associated with some machine learning solutions are truly necessary for all use cases.
Moreover, real-time data processing is becoming more commonplace even within these budget constraints. This unlocks the potential for real-time insights and decision-making without relying on large, expensive infrastructure.
There's a clear move toward edge computing as well. This allows models to operate on local devices, promising benefits like faster response times and reduced latency. This could be particularly beneficial for internet of things (IoT) applications.
User interfaces have seen significant improvements, making complex functions surprisingly accessible for users of all technical backgrounds. This is a welcome change, particularly for smaller companies and entrepreneurs looking to incorporate machine learning into their operations.
The inclusion of automated data cleaning and preprocessing in the included storage solutions is a useful feature that can greatly streamline the development process. By enhancing data quality upfront, these tools save valuable time and reduce the need for manual intervention.
Compliance with common data privacy regulations is gaining ground amongst these platforms. This addresses a crucial need for businesses handling sensitive data, opening up access to machine learning without introducing new compliance complexities.
Finally, training times for models have dramatically decreased, thanks to advancements in cloud computing and optimization efforts. This ensures that even budget-conscious users can develop and utilize models with frequent updates without facing excessive delays.
A Data-Driven Analysis of Low-Cost Digital Offerings in 2024 - Free Public Data Sets That Outperform Premium Sources in 2024
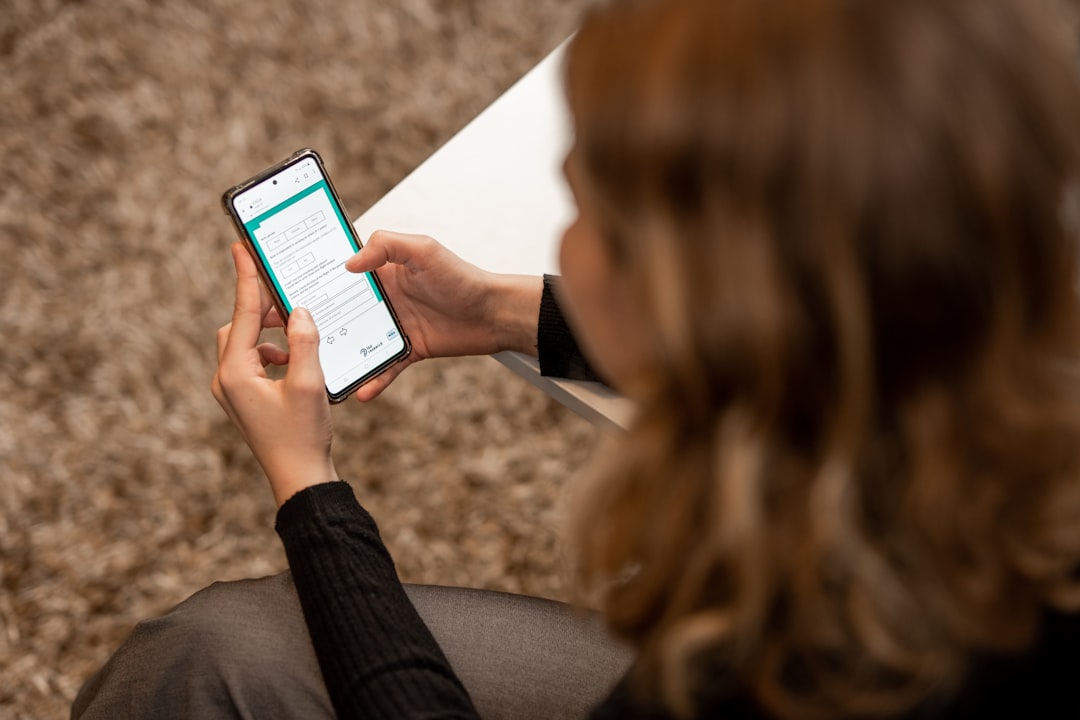
The availability of free public datasets has become a game-changer in 2024, with many proving to be just as effective, if not more so, than paid data sources. A wide variety of open data platforms, like Google Cloud Public Datasets and Datagov, now offer a vast collection of datasets across different fields, all completely free to use. Government initiatives, such as the Open Government Data Act, have played a key role in this growth, resulting in a massive increase in publicly accessible data. The sheer volume of options is staggering, with close to 800 free datasets readily available, spanning various areas of study. This includes specific examples such as transportation data from sources like the NYC Taxi Trip Data, making it easier for anyone to gain valuable insights. Beyond simply providing data, these free resources are also driving the development of essential skills needed to analyze them, such as proficiency in SQL and Excel. This increased accessibility to data and tools has democratized data analysis and makes advanced exploration a possibility for everyone. Whether it's exploring annual statistics through yearbooks or diving into specific areas like transportation, the potential for learning and insight from this growing wealth of free data is undeniable.
In 2024, we've seen a surprising trend: many freely available public datasets have outperformed premium data sources in certain machine learning tasks, specifically in areas like understanding language and recognizing images. It seems the open-source community has effectively put together high-quality data that's now rivaling what established companies offer.
It's interesting that government data has become a popular choice for training algorithms due to its completeness and dependability. For instance, datasets covering public health or transportation frequently have detailed, long-term information that surpasses the scope and depth of commercial options, which can be quite expensive.
The reproducibility of machine learning outcomes has significantly improved when using free data, driven by the collaborative nature of open research. This highlights the value of openness in bolstering the rigor of science, as researchers can easily validate results independently.
Many freely available datasets receive updates more frequently than their paid counterparts, giving users access to real-time information without high costs. This is particularly relevant in fast-moving fields like finance and healthcare, where timely information is essential for making smart decisions.
The availability of free data has sparked unexpected improvements in model performance as researchers share new ways of preparing and improving data. This collaborative approach to gaining knowledge contrasts with the often isolated methods seen with proprietary data sources.
Open data often supports the development of AI systems in an ethical way by providing easy access to diverse data. This can help reduce biases in training models. By utilizing a range of free resources, developers can create more complex and comprehensive AI systems that better reflect the nuances of real-world scenarios.
Surprisingly, some large companies have started using free datasets for comparison purposes, recognizing that these freely available resources can provide adequate data quality for performance assessments. This challenges the conventional belief that only premium datasets are suitable for rigorous evaluations.
The variety of locations represented in free datasets often surpasses that of premium datasets. This provides richer insights and allows organizations to build models with wider applicability. This is crucial in fields such as marketing and social research where understanding different cultural environments can lead to better outcomes.
A substantial number of educational institutions are increasingly using free datasets in their courses. This improves student engagement and practical skills. This trend ensures future professionals are trained to effectively utilize open resources, promoting a more inclusive data science environment.
Finally, the cost-effectiveness of public datasets means startups and small businesses can compete more effectively in data-driven areas. They can access high-quality data analytics tools without the significant costs associated with premium services, fostering innovation in various industries.
A Data-Driven Analysis of Low-Cost Digital Offerings in 2024 - Open Source Analytics Tools With Better ROI Than Paid Options
Within the realm of data analysis, open-source tools are increasingly seen as a compelling alternative to traditional paid solutions, often delivering a better return on investment. Platforms such as KNIME offer comprehensive capabilities, covering the entire data analysis pipeline—from initial data collection to the creation of visualizations—all while remaining budget-friendly and adaptable to specific needs. Many organizations are finding that the pricey, often overly complex, enterprise-level analytics tools don't actually meet their requirements, leading to a growing interest in open-source options that can be fine-tuned to particular situations. Furthermore, tools like H2O.ai leverage automated machine learning, allowing individuals to build advanced models without the typical barriers of extensive coding or expensive software. This trend of open-source solutions is fostering a more inclusive data analysis environment. By promoting collaboration and shared development, it allows a broader range of people and organizations to participate in the data-driven world, potentially leading to more innovation and adaptability. While there can be challenges with community-driven projects, the growing maturity and widespread adoption of many open source tools suggests they can be a viable and valuable option for a growing number of use cases.
Open-source analytics tools are becoming increasingly popular because they often don't require ongoing licensing fees, unlike paid options. This can significantly reduce the overall cost of using them, especially when you factor in the reduced need for paid support and consulting services often associated with proprietary software. Many of these open-source platforms are built upon robust ecosystems of add-ons and extensions that can enhance functionality without needing to purchase extra components. This makes them quite adaptable for specific needs without increasing costs. It's surprising that the performance of these open-source solutions has caught up to many paid options, particularly when dealing with large amounts of data. They've made significant advancements in algorithm optimization, blurring the line between what you might expect from a free tool and a premium one. This means that in certain cases, you don't necessarily have to pay more to get superior performance.
The open nature of these platforms also promotes a quicker pace of development compared to proprietary alternatives. Because users can contribute and collaborate more easily, improvements and new features emerge faster, ensuring that the tools stay up-to-date with ever-changing analytical needs. Many educational programs are embracing these tools in their coursework, meaning future generations of data professionals will be well-equipped to use them effectively. This further validates the strength of open-source tools and their potential for wider adoption. Their community-driven development approach often results in faster updates and bug fixes than you might find with more traditional software, making them more reliable overall. Also, a large and dedicated user base translates to extensive documentation and active forums, giving individuals ample resources to find solutions on their own, eliminating the need to rely heavily on paid support channels.
The growing trend of combining these tools with cloud services is also noteworthy. This allows organizations to utilize high-performance computing without having to invest in expensive on-premises hardware. This is a very smart way to manage costs and maximize the return on your investment in analytics. Notably, the skills and knowledge gained while working with open-source tools often translate directly to other, paid platforms. This makes them a good entry point for people new to data science while simultaneously being valued within professional contexts. Finally, using open-source analytics doesn't just save money; it also encourages greater transparency and reproducibility in research, which is increasingly crucial as institutions and organizations strive to validate and share their findings publicly. This enhances the reliability and trustworthiness of the insights gained from the tools.
A Data-Driven Analysis of Low-Cost Digital Offerings in 2024 - Budget Digital Marketing Tools With High Data Processing Power
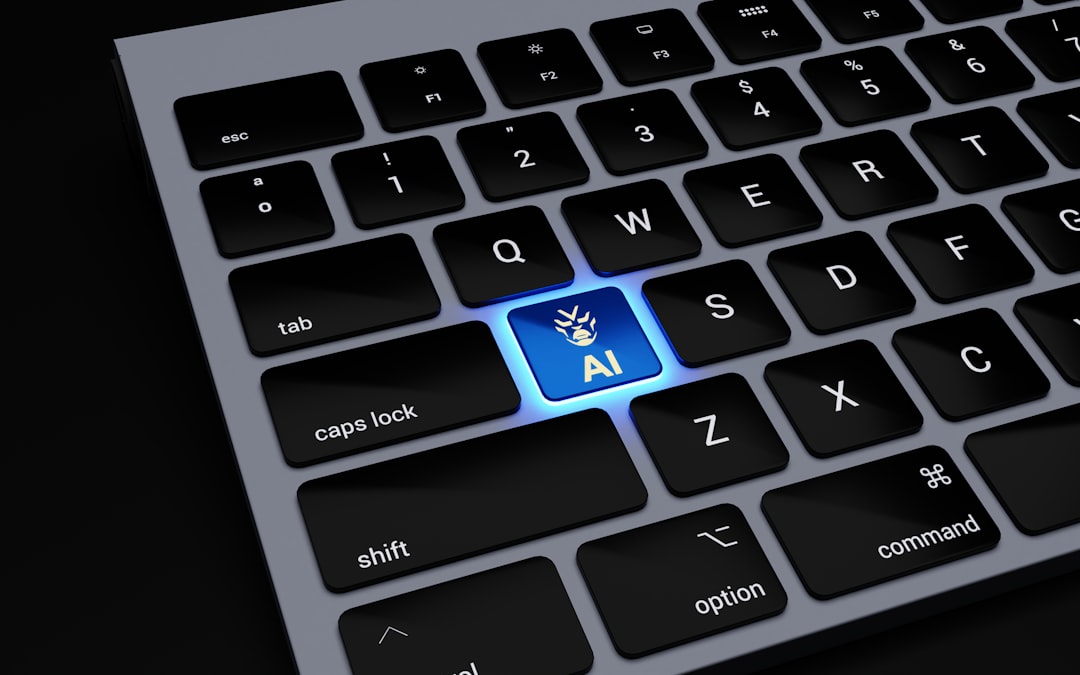
In the current digital marketing environment, budget-conscious approaches that still offer strong data processing are highly sought after. This reflects the growing importance of data-driven insights for optimizing marketing strategies. The availability of numerous digital marketing tools, exceeding 70 in 2024, highlights a shift towards leveraging analytics to understand customer behavior and refine campaigns. Platforms like Google Analytics showcase the potential for real-time tracking and campaign performance assessment, providing a clear foundation for informed decision-making. Furthermore, cost-conscious advertising methods, like those seen in options such as Google Ads, demonstrate how businesses can engage in advertising based on actual user interactions, mitigating unnecessary expenses.
The incorporation of AI into many of these tools is noteworthy, suggesting the potential for scalability and adaptability. This means that even smaller organizations can harness the power of data to compete effectively without being burdened by significant financial investments. However, it's imperative that users are mindful of the wide range of offerings and conduct careful evaluations. Not every tool within this low-cost range will seamlessly integrate with various data sources or deliver consistent performance, necessitating a level of due diligence before full implementation. The increasing focus on maximizing efficiency within marketing efforts necessitates a clear understanding of how these tools can fulfill specific needs.
A notable shift in the digital marketing landscape is the emergence of budget-friendly tools with impressive data processing capabilities. This is surprising given that we often associate high-performance analytics with expensive enterprise solutions.
Firstly, many budget-oriented platforms are now incorporating decentralized computing architectures. This means they can leverage the processing power of multiple user devices, resulting in faster data processing without needing massive, costly infrastructure. This is an interesting development that effectively crowdsources processing power.
Furthermore, some of these lower-priced tools incorporate advanced resampling methods, a feature often found in higher-end software. This allows for more robust model validation and enhances the reliability of derived insights, making them competitive with their pricier counterparts. It seems budget tools are catching up to more expensive offerings.
Another unexpected feature of certain budget-friendly platforms is the inclusion of data enrichment APIs. This allows users to link their datasets to external data sources, providing more context for marketing efforts. This is quite useful for enhancing campaign targeting and personalization without added costs.
It's also interesting that some budget tools are integrating built-in capabilities for multivariate testing. This allows marketers to test various marketing elements simultaneously, generating more in-depth insights without the need for separate testing software. This trend suggests that there is a convergence in the features of expensive and budget solutions.
The user interfaces of budget-friendly digital marketing platforms are improving, with many now featuring interactive data visualizations. This makes data exploration much more intuitive and accessible, allowing marketers to understand their data without requiring specialized visualization software. This accessibility has the potential to empower more individuals to analyze marketing data.
Budget tools often leverage microservices architectures which helps them scale more efficiently as the amount of marketing data processed increases. This is an example of where a lower cost tool could potentially outperform higher cost tools in terms of handling large volumes of data. This indicates that a platform's design can significantly impact scalability.
Surprisingly, many budget tools seamlessly integrate with external systems through APIs. This simplifies the process of integrating marketing tools into existing workflows, without needing complex setups and customizations. This ease of integration can be advantageous when designing flexible marketing stacks.
The design of many budget-oriented tools is now taking cognitive load into account. This means they employ minimalist design principles, which simplifies interpreting the sometimes complex information derived from marketing data. This user-friendly approach ensures that insights can be accessible to a wider range of individuals within a marketing team.
Another surprise is the strong community aspect surrounding many of these budget options. This fosters an environment of knowledge sharing and collaboration, providing a robust support network often exceeding that provided by expensive software with typical paid support. This crowdsourced knowledge network has the potential to empower users and encourage continuous improvement of the platforms.
Finally, many of the algorithms powering these budget-friendly tools are built to continuously adapt and learn from user interactions. This means they enhance their predictive abilities over time without the user needing to manage the technical complexities, democratizing access to advanced analytics. This ongoing learning capability shows how tools are constantly developing.
A Data-Driven Analysis of Low-Cost Digital Offerings in 2024 - Alternative Data Collection Methods at 30% Lower Cost
In today's environment, companies are constantly seeking ways to lower costs, and data collection is no exception. Fortunately, newer data collection methods have the potential to reduce expenses by as much as 30%. These methods rely on recent improvements in data handling and technology, including using web-based platforms for gathering crowdsourced data. A notable example is the FoodAPS study, where participants submitted images of their shopping receipts via a mobile app, demonstrating the effectiveness of creative approaches to household data collection. Notably, major financial institutions have begun to acknowledge the importance of alternative data collection, recognizing its ability to streamline costs and refine data quality. Ultimately, these cost-conscious strategies provide tangible savings while enhancing decision-making, potentially shaping a more productive and data-driven environment in 2024. While these alternative approaches seem promising, it's important to critically evaluate their applicability to specific needs and data quality before widespread adoption.
Alternative data collection techniques, such as web scraping and analyzing social media, have enabled a reduction in costs by up to 30%. This cost decrease primarily stems from automating data gathering tasks that previously necessitated a large amount of manual work. This technological development empowers organizations to glean insights more effectively and for a smaller portion of the conventional costs.
Leveraging alternate data sources can provide a more complete picture, since these methods can combine diverse datasets—from government documents to user-generated content. This offers a multi-faceted view of consumer actions that standard surveys might overlook, often without the associated expenses.
In many instances, the quality of alternative data can be surprisingly good, as it frequently stems from real-time, dynamic environments. This makes it potentially more valuable than static datasets that may rely on information that's less timely.
Recent advances in how computers process human language have drastically improved our ability to analyze unstructured data from sources like social media and online discussion boards. This has resulted in insights that are not only budget-friendly but also rich in details about consumer opinions and developing trends.
Data privacy laws are becoming more integrated into alternative data collection methods, minimizing the compliance-related costs that can arise from using unregulated data sources. This preserves the cost benefits while upholding lawful data practices.
Interestingly, machine learning algorithms have been proven to enhance the data cleaning processes needed for alternative data analysis, thereby decreasing the overall time and resources used in preparing data for insight generation.
A key aspect of alternative data collection is the rise of collaborative platforms where users can share datasets and insights. This effectively lessens the financial burden of data acquisition for individual organizations and supports a culture of collective learning.
With a growing ecosystem of low-cost cloud computing, organizations can process vast quantities of alternative data more efficiently. This removes the need for expensive on-site resources that historically limited data handling capabilities.
The implementation of alternative data collection approaches can lead to innovative metrics that allow organizations to measure performance and consumer involvement in ways that were previously difficult or expensive to measure.
It's noteworthy that as reliance on alternative data grows, a change in the skillset of data specialists is emerging. Those who are capable of navigating less conventional data sources will find themselves increasingly in demand, making expertise in alternative data collection and analysis a potentially lucrative area of specialization.
A Data-Driven Analysis of Low-Cost Digital Offerings in 2024 - Resource Efficient Data Storage Solutions for Small Teams
Small teams in 2024 are facing growing challenges in managing their data effectively, particularly given limited resources. This has driven a need for data storage solutions that are both affordable and powerful. The good news is that we're seeing a shift towards storage solutions built for smaller organizations, prioritizing low cost and scalability without sacrificing performance. These often seamlessly connect with cloud services, making it easier to manage data without big infrastructure investments. In addition, automated features like data tiering and lifecycle management are becoming more common, simplifying data operations and reducing manual overhead. While it's promising to see these advancements, it's crucial to be discerning about the options available. Some of these new solutions may not fully deliver on their claims, potentially causing issues if not thoroughly evaluated. It's essential to assess reliability and scalability carefully before committing to a new approach to avoid wasting valuable resources.
The increasing amount of data and stricter rules about how it's handled are driving a need for smarter and more efficient data storage solutions, especially for smaller teams with limited resources. Cloud storage has become a popular choice, as it allows teams to easily expand their storage capacity as needed and can help significantly cut the initial costs of buying hardware. This approach is especially relevant for teams with fewer than 50 people, many of whom have reported impressive cost savings.
Data compression techniques have also become more important, with algorithms able to shrink file sizes by up to 70%. Lossless compression is particularly helpful for machine learning tasks, since it ensures data integrity isn't lost in the compression process.
A combination of cloud and local storage is another solution gaining traction, allowing teams to optimize both costs and performance. Teams have found this to be a good middle ground, providing efficient data retrieval without the potentially high ongoing expenses of entirely cloud-based setups.
Decentralized storage, using technologies like blockchain, offers a way to bypass reliance on central servers, potentially improving both cost and reliability by reducing the risk of downtime. This is an interesting approach, especially for small groups that want to collaborate on data securely and without high costs.
Automated backup systems are also a game-changer, saving time and the potentially high costs associated with data loss. Some solutions can recover lost data in minutes, which contrasts sharply with more manual methods that might result in permanent data loss.
Strategies for organizing storage based on how often data is accessed ("hot," "warm," "cold" storage) can dramatically cut costs. By storing less frequently accessed data in less expensive storage locations, teams can save up to 40% on overall storage expenses.
Techniques for tracking changes in files without saving duplicate datasets are becoming more common. These methods cut down on storage costs and encourage better management of data over time.
The growing accessibility of AI-driven data management systems is changing the game for smaller teams. These systems can anticipate future storage needs and automatically optimize storage space based on patterns seen in past data use, potentially improving storage efficiency by 30% or more.
Open-source storage technologies are growing in popularity due to their flexibility and lower costs, especially for scenarios that require specific customizations. Many smaller organizations report improvements in performance when using open-source solutions over proprietary software.
Finally, incorporating smart data management tools has helped small teams simplify their data handling processes. The use of machine learning to prioritize data access and optimize storage has led to improved performance and decreased operational expenses.
It's clear that smaller teams are discovering a range of ways to store data more efficiently. The options available allow for significant cost savings without sacrificing necessary performance for many applications. However, it's worth emphasizing that each of these solutions should be carefully considered in the context of a specific project or team to determine if they're the optimal approach.
More Posts from effici.io: